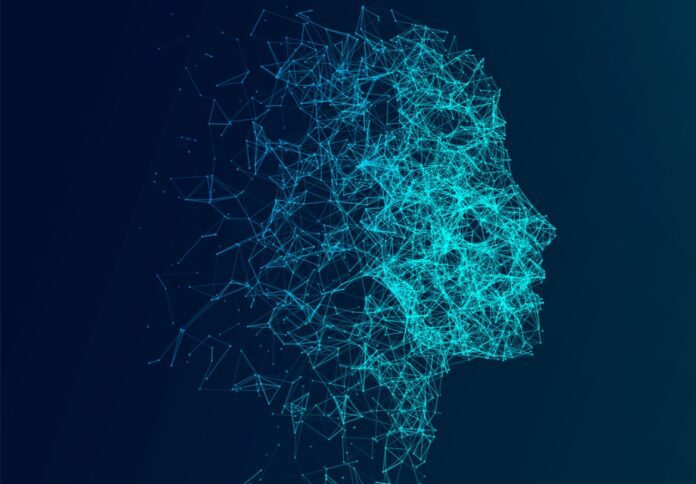
Researchers have developed a new artificial intelligence algorithm, Torque Clustering, which they claim brings AI closer to natural intelligence by enabling systems to learn and identify patterns independently, without human intervention.
The research team, led by academics from the University of Technology Sydney (UTS), said the algorithm significantly improves the way AI processes vast amounts of data autonomously.
According to Distinguished Professor CT Lin from UTS, the development aligns with the principles of unsupervised learning, which differs from the widely used supervised learning methods that require extensive labelled data.
“In nature, animals learn by observing, exploring, and interacting with their environment, without explicit instructions. The next wave of AI, ‘unsupervised learning,’ aims to mimic this approach,” Lin said.
Supervised learning, which currently dominates AI applications, relies on predefined categories assigned by human annotators, a process that can be costly and impractical for large-scale or complex tasks.
In contrast, Torque Clustering identifies inherent patterns in datasets without requiring prior labelling, making it a potentially more efficient alternative.
The research findings were recently published in IEEE Transactions on Pattern Analysis and Machine Intelligence, a leading journal in artificial intelligence.
According to first author Dr Jie Yang, the method stands out due to its basis in the physical concept of torque, which allows it to identify clusters autonomously while adapting to various data structures.
“What sets Torque Clustering apart is its foundation in the physical concept of torque, enabling it to identify clusters autonomously and adapt seamlessly to diverse data types, with varying shapes, densities, and noise degrees,” Yang said.
The researchers said the algorithm had been rigorously tested across 1,000 diverse datasets, achieving an average adjusted mutual information (AMI) score of 97.7 per cent, surpassing the performance of other state-of-the-art clustering methods that typically achieve scores in the 80 per cent range.
They also noted that Torque Clustering is fully autonomous, parameter-free, and computationally efficient, which could make it more scalable for real-world applications.
Yang explained that the approach was inspired by the torque balance in gravitational interactions when galaxies merge, drawing on natural physical properties such as mass and distance.
He also compared the significance of unsupervised machine learning to last year’s Nobel Prize-winning advancements in supervised machine learning with artificial neural networks.
The research team believes the method could contribute to the development of general artificial intelligence, particularly in robotics and autonomous systems, by improving movement optimization, control, and decision-making capabilities.
The open-source code for the algorithm has been made available to researchers, allowing for further study and potential applications in various domains.