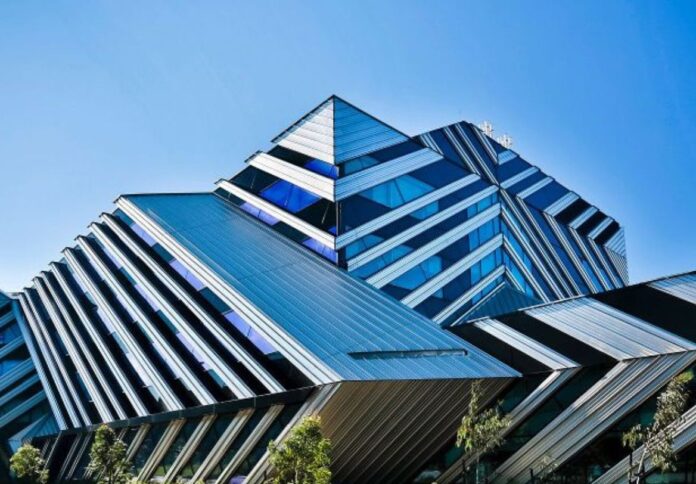
Australian researchers, led by Monash University, have developed an artificial intelligence (AI) tool poised to transform virtual screening in early-stage drug discovery.
This innovation, known as PSICHIC (PhySIcoCHemICal), aims to enhance scientists’ ability to identify potential new medicines efficiently and economically, the university said in a news release.
Dr Lauren May, co-lead author from the Monash Institute of Pharmaceutical Sciences (MIPS), mentioned that the team has successfully shown PSICHIC’s capability to screen new drug candidates and conduct selectivity profiling effectively.
“Comparison of experimental and AI predictions of a large compound library against the A1 receptor – a potential therapeutic target for many diseases – demonstrated PSICHIC’s effectiveness,” Dr May added.
“Moreover, PSICHIC was able to distinguish the functional effects of the compound or, in other words, the way in which the drug might affect our bodies.”
Published in Nature Machine Intelligence, the study illustrates how PSICHIC leverages sequence data and AI to decode protein-molecule interactions with cutting-edge accuracy, bypassing the need for expensive and less precise methods like 3D structures.
“The application of AI approaches to enhance the affordability and accuracy of drug discovery is a rapidly expanding area,” noted Professor Geoff Webb, lead author from Monash’s Department of Data Science and Artificial Intelligence.
Webb added, “With PSICHIC, our team has eliminated the need for 3D structures to map protein-molecule interactions, which is a costly and often restrictive requirement.”
Dr Anh Nguyen, co-lead author from MIPS, emphasised the significance of understanding these interactions. “Drugs exert their effects by selectively interacting with specific proteins. Accurately predicting these interactions is crucial for developing new medicines,” he said.
Huan Yee Koh, a PhD candidate and first author from Monash’s Faculty of Information Technology, explained the motivation behind PSICHIC’s design.
“AI has the potential to dramatically improve the robustness, efficiency and cost at multiple stages during the drug discovery process, from early stage discoveries right through to predicting clinical responses,” Koh noted.
He continued, “However, since many AI systems fundamentally rely on pattern matching, these systems can suffer from unrestrained degrees of freedom. This can lead to memorisation of previously known patterns rather than learning the underlying mechanisms of protein-ligand interactions, ultimately hindering the discovery of novel drugs.”
Professor Shirui Pan, co-lead author and ARC Future Fellow at Griffith University, highlighted the tool’s accessibility.
“Compared to previous deep sequence-based methods, this approach provides a more faithful representation of the underlying protein-molecule interactions, thereby closing the performance gap between sequence-based methods and structure-based or complex-based methods.”
The full study, titled Physicochemical graph neural network for learning protein-ligand interaction fingerprints from sequence data, can be read here.